KANSAS CITY, MISSOURI, US — The closing sentence of my article in the April 2023 issue of World Grain was: “You cannot manage what you cannot measure.” It often is attributed to W. Edwards Deming, the statistician and quality-control expert credited with having launched the Total Quality Management (TQM) movement, and at other times it is attributed to Peter Drucker, perhaps the world’s most famous management consultant ever.
Others have suggested that neither made such a statement as it is too simplistic and misleading. As Deming points out, nothing becomes important just because it can be measured, especially with little or no consideration of other data that is unknown or unknowable. From a milling perspective, the benefit of uniform tempering or conditioning is well known and appreciated.
However appreciated the process of tempering or conditioning is, its management and control often is compromised by the lack of data, improper interpretation of data, and human intervention, whether the conditioning system is manually controlled or automated. Manually controlled and monitored systems utilize limited data collection and analysis procedures often responding by making an adjustment and operating for a period before the next monitoring or sampling time. Between sampling periods, a significant amount of wheat passes through the system with some level of inherent moisture and flow rate variability. For example, in a 300-tonne-per-day day mill (approximately 5,000 cwt/day flour) 12.5 tonnes/hour of wheat is tempered.
On a per-minute basis, the wheat rate is significant at 208 kg/minute, 459.3 pounds/minute or 7.6 bushels/minute. Undoubtedly there is much that is unknown and unknowable about such a process such as the dry wheat flow rate and wheat moisture. The assumption of stability in dry wheat moisture content, dry wheat flow rate and water meter setting is not correct and is further compromised when tempered wheat moisture taken at B1 is used to adjust moisture addition.
An individual moisture measurement is a spot observation of moisture content for a sample. Within a static 250-gram sample of wheat, an NIR taking 10 moisture measures may report a difference between the maximum and minimum moisture content of the 10 measurements as large as 0.5%, while the average difference across 50 or more samples is 0.2% or less. Clearly, moisture measurement within any wheat mass, no matter how small the sample, is inherently variable.
A single measurement ignores that variability and prevents us from understanding from what average value might the single observed moisture measurement represent. To provide a meaningful understanding and, hence, ability to manage the tempering process we must understand both the average and variability or standard deviation of the moisture measurement observations. More importantly, we should respond only to changes or movement in the average moisture value, which moves us away from our target value or set point as well as changes in measurement variability.
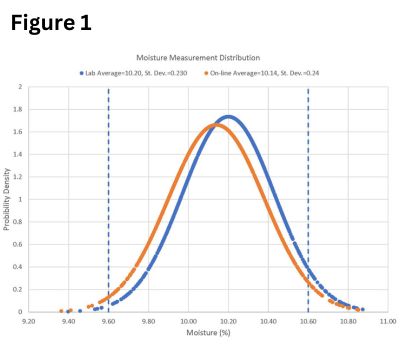
Moisture measurement distribution using two different measurement methods.
| Credit: ©JEFF GWIRTZAnalyzing the data
Figure 1 compares two sets of moisture data for dry wheat using normal distributions based on nine measurements made using a Lab NIR and an online measuring device. In the graphic, the average and standard deviation are provided for each measurement system based on N=1500 observations. The mean value reported differs by 0.06% and the standard deviation, a measure of variability, is quite similar for both moisture measurement methods, averaging 0.235%. The moisture measurement distributions are neither statistically nor practically significantly different. The 95% Confidence Interval for individual moisture observations is approximately 10.2± 0.5%, while the 95% Confidence Interval for the mean value is 10.2± 0.01%.
Sample measurements may vary between 9.6% to 10.6% within the same distribution whose average value is 10.2%. Adjusting moisture addition based on a single observation would not only be unwarranted but likely increase variability and widen the Confidence Interval about the mean value. Intervention is not warranted based on a single observation period.
The ability to collect and analyze moisture data in a manual operation is undoubtedly a challenge. One might start with taking multiple samples, perhaps N=5 as might be done on a packaging line to monitor packaging line performance and record the average moisture and range between the minimum and maximum moisture value measured. Respond only to multiple consecutive increasing or decreasing changes in moisture measurement before adjusting water addition. Changes in the moisture range may reflect blending uniformity or bin changes that should be explored. Variability is endemic and will be observed. However, every change does not warrant adjustment.
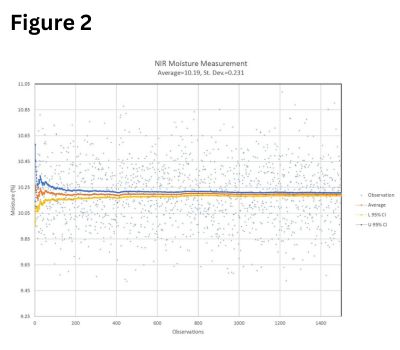
NIR moisture measurement observations, average and 95% confidence interval dry wheat.
| Credit: ©JEFF GWIRTZIndividual observations, the average, and a 95% Confidence Interval for NIR moisture measurement of dry wheat is shown in Figure 2. As can be observed, the average values and confidence interval fall in a much narrower band than the individual observations. Imagine the frustration and extra work it takes to respond to single observations. Moreover, the distribution of individual observations would become much wider as would the confidence interval about the mean or average value. With limited observations, the impact would be even greater.
Figure 3 provides the average and a 95% Confidence Interval for NIR moisture measurement of dry wheat early observations shown in Figure 2. Given limited data points the estimate of the mean value varies more wildly as does the Confidence Interval for the mean value.

NIR moisture measurement observations, average and 95% confidence interval dry wheat, the early estimates based on limited number of observations.
| Credit: ©JEFF GWIRTZAs can be observed beyond 30 to 40 observations, the estimated mean and Confidence Interval become more stable. Beyond this point, the average value and the 95% Confidence Interval variability reflects the inherent variability of the dry wheat mix rather than the impact of relying on limited sample data for the estimate of the average value its 95% Confidence Interval. As some may recall, N=30 is the trigger point for statistical analysis using sample statistical analysis (t-score) and population statistical analysis (Z-score). Repeated measures improve the calculated population mean and standard deviation estimate, providing a more reasonable assessment of product or system variability.
The ability to collect and analyze data manually is challenging, especially with scheduling of short grist or mill mix runs. Leveraging data collection and analysis across cumulative short runs of a given grist or mill mix may be a useful approach. Automation may allow for increased data collection, analysis, and most importantly on-screen reporting. Imagine a tempering process control system reporting real time data for dry wheat flow rate, dry wheat moisture and water addition rate and estimated tempered wheat moisture while considering any programed “offset.”
To avoid the introduction of variation beyond the inherent variation of the tempering system, carefully consider the program of managing your automated tempering system with limited manual measurement. If the process is to be controlled, it is not simply a matter of taking measurements, it is taking the correct measurements sufficient to reflect the nature of the process. Only when sound measures are available should intervention be undertaken to manage the process.
Jeff Gwirtz, a milling industry consultant, is president of JAG Services, Inc. He may be reached at [email protected].